Incident 535: COVID-19 Detection and Prognostication Models Allegedly Flagged for Methodological Flaws and Underlying Biases
Description: Peer-review of papers about COVID-19 detection and prognostication algorithms from 2020, including deployed models, revealed none to be ready for clinical use, due to methodological flaws and underlying biases such as lacking external validation or not specifying data sources and model training details.
Entities
View all entitiesAlleged: unknown and Icahn School of Medicine researchers developed an AI system deployed by Mount Sinai Hospital and unknown, which harmed COVID-19 patients and COVID-19 healthcare providers.
Incident Stats
Incident ID
535
Report Count
2
Incident Date
2020-01-01
Editors
Khoa Lam
Incident Reports
Reports Timeline
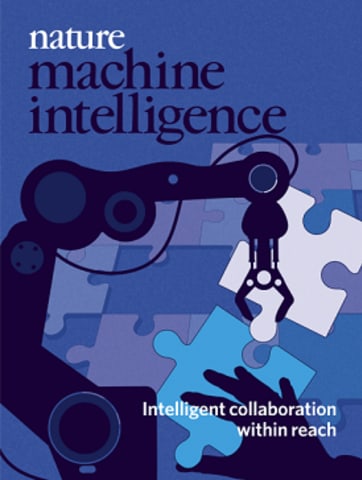
nature.com · 2021
- View the original report at its source
- View the report at the Internet Archive
Abstract
Machine learning methods offer great promise for fast and accurate detection and prognostication of coronavirus disease 2019 (COVID-19) from standard-of-care chest radiographs (CXR) and chest computed tomography (CT) images. Many a…
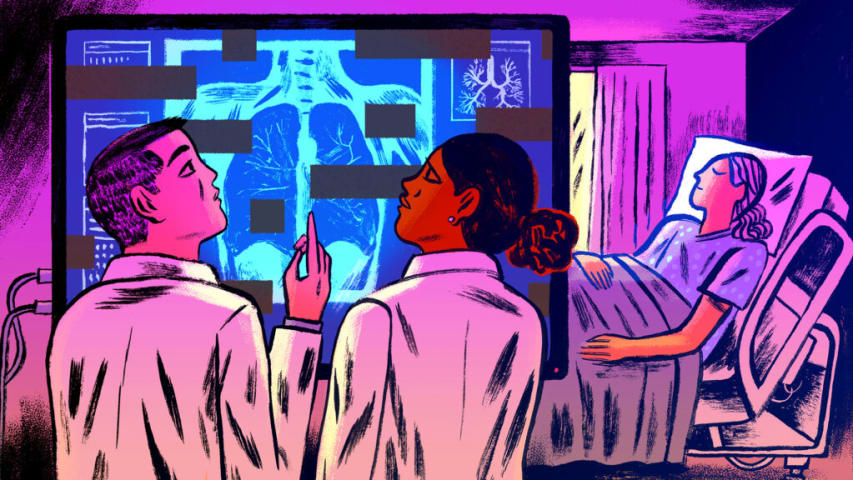
statnews.com · 2021
- View the original report at its source
- View the report at the Internet Archive
The mad dash accelerated as quickly as the pandemic. Researchers sprinted to see whether artificial intelligence could unravel Covid-19's many secrets — and for good reason. There was a shortage of tests and treatments for a skyrocketing nu…
Variants
A "variant" is an incident that shares the same causative factors, produces similar harms, and involves the same intelligent systems as a known AI incident. Rather than index variants as entirely separate incidents, we list variations of incidents under the first similar incident submitted to the database. Unlike other submission types to the incident database, variants are not required to have reporting in evidence external to the Incident Database. Learn more from the research paper.